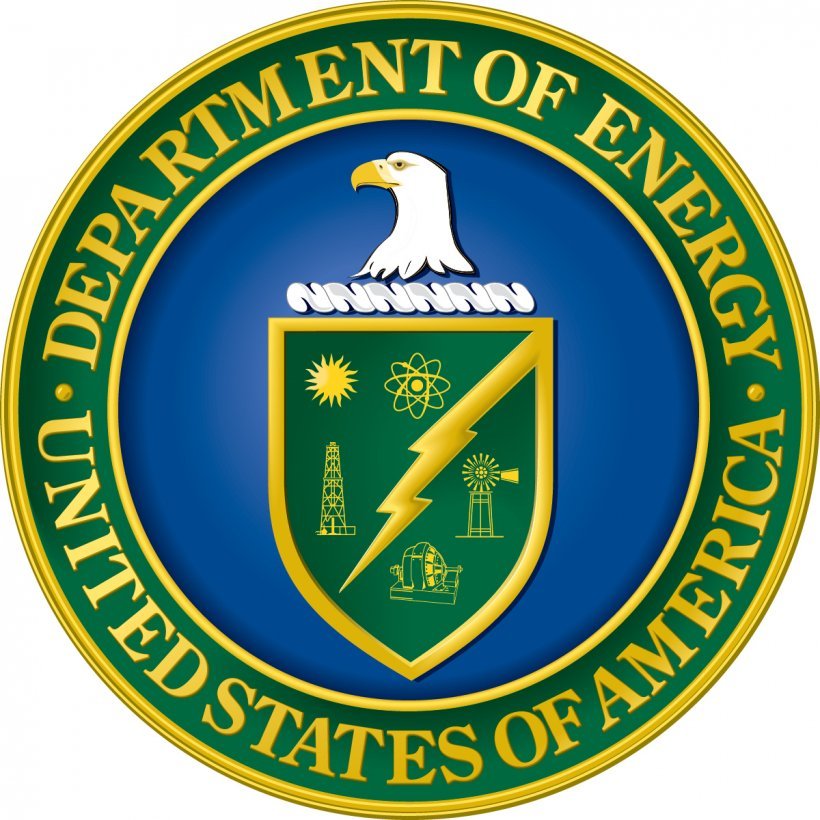
Quantum Computing and Quantum Machine Learning
Duration: February 1, 2023 – December 31, 2025
Total Award Amount: $46,000
Investigator(s): Arslan Munir (KSU)
Sponsor: Kansas State University, Computer Science Department and Florida Atlantic University, Electrical Engineering and Computer Science Department
Project Abstract
Quantum computing has emerged as a new field of scientific research and development that exploits the synergy between quantum mechanisms and computer science. Quantum computing has stirred a burgeoning revolution in the fields of computing and machine learning. Although quantum computers have some of their components named similar to those of classical computers, such as registers, gates, and memory elements, their underlying physical structures are fundamentally distinct and unique. Quantum computers operate on qubits (the quantum counterpart of classical bits) that are capable of existing in states of zero, one, or any intermediate value and exhibit superposition and entanglement properties. These unique attributes empower quantum computers to simultaneously follow multiple computational paths within a single calculation, which is not possible by classical computers without repeated iterations. Quantum computing is capable of enhancing the machine learning design process due to its ability to speed up linear algebraic operations exponentially as state space grows. With the advent of noisy intermediate-scale quantum (NISQ) processors, quantum machine learning based on heuristic methods has gained momentum due to the increased computational capabilities of quantum hardware, particularly in the field of deep learning. Since quantum processors are still fairly small and noisy, to improve machine learning performance effectively, NISQ processors often work with classical co-processors in hybrid mode, giving rise to hybrid quantum–classical machine learning.
This project targets advances in quantum computing and quantum machine learning. Topics of interest to this project include, but are not limited to, quantum computing, quantum machine learning, hybrid quantum-classical computing, hybrid quantum-classical machine learning, noisy intermediate-scale quantum (NISQ) processing, circuits for quantum computing and machine learning, performance analysis of classical versus quantum computing, hybrid quantum-classical deep learning, hybrid quantum-classical neural networks, and applications of (hybrid) quantum computing and machine learning.
Publications
- Muhammad Asfand Hafeez, Arslan Munir, and Hayat Ullah, ” H-QNN: A Hybrid Quantum-Classical Neural Network for Improved Binary Image Classification”, under review in AI, 2024. Download
- Muhammad Ali Shafique, Arslan Munir, and Imran Latif, “Quantum Computing: Circuits, Algorithms, and Applications”, IEEE Access, vol. 12, pp. 22296-22314, February 2024. Download